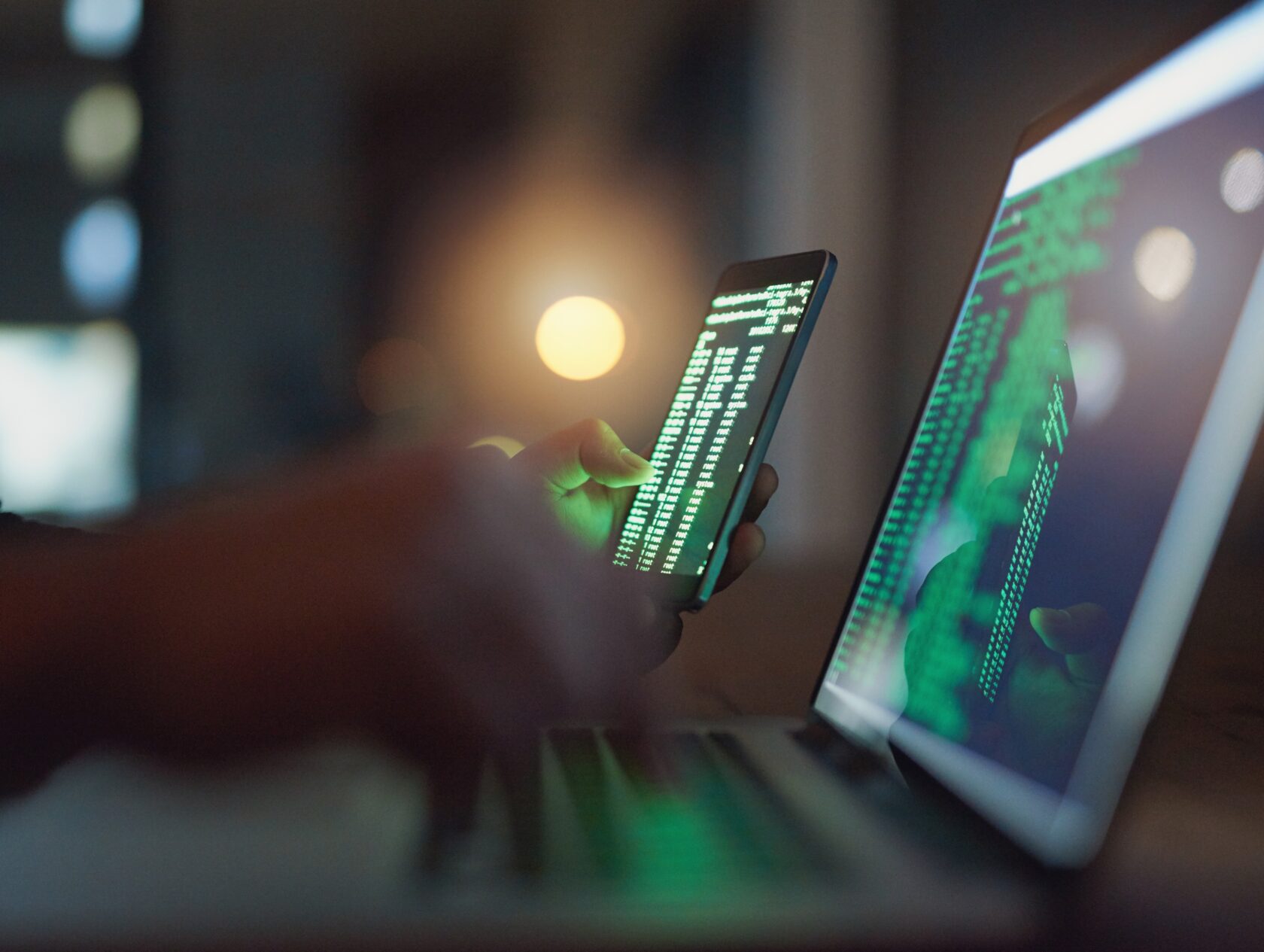
Trusting AI: Threat Detection in SIEM Tooling
Ad tempus
It’s about to become 2025 and the world continues to change. Businesses look to evolve and adopt advanced technologies, the concept of trusting AI becomes an essential consideration. When it comes to Security Information and Event Management (SIEM) tooling, the shift from human-written threat detections to AI-driven mechanisms marks a significant transformation. While human-crafted detections provide predictability and control, AI-based systems automate adaptability and anomaly-focused insights. However, this shift raises critical questions about reliability, accuracy, and how trust in AI shapes the future of cybersecurity.
AI Informed
Hand on heart honesty here. The original draft for this blog post was written by AI. Just like threat detection writing, it got most of the context right, whilst also being very wide of the mark on some very key aspects. Would I trust AI to write a whole blog article for me – no. Do I need a method to test the AI output – absolutely. Do I suspect that AI generates content that favours AI over human generation – completely.
If I can't trust AI to write something like this blog post, what kind of business would be trusting AI to look after its Crown Jewels ?
Now it could be a business that can’t acquire the skilled threat developers it needs. It could also be someone who has put their complete faith into the marketing team of the reseller. Either way, there is a need to educate that trusting AI is ‘okay’, but you have to understand that you need to apply your own governance and assurance over its use.
The Role of Human Expertise in Threat Detection
Traditionally, threat detection in SIEM tooling relies on human expertise. Data scientists and Security analysts craft rules and logic tailored to specific attack vectors.
For a very old style example, if detecting brute force attempts on a login portal, the analyst might define a rule to trigger alerts after a set number of failed login attempts with correlations with other data sets within a certain timeframe.
Human-written detections excel in their clarity and repeatability. When the detection logic is deployed, security teams can inject real or test data and confidently expect the system to flag the intended activity. This predictability allows for straightforward validation and fine-tuning, creating a sense of assurance that the detection operates as intended.
However, this approach sometimes has limitations:
- Static Nature: Human-crafted rules are often static and may not adapt to evolving attack techniques.
- Overhead: Writing and maintaining rules can be resource-intensive, requiring significant time and domain expertise.
- Blind Spots: Analysts may not foresee every potential attack vector, leaving gaps in detection. Notably here though, these blind spots are more about missing data – rather than missing detections. Even AI cannot detect when data is not present.
These limitations highlight the need for more dynamic and adaptive threat detection solutions—a need that AI promises to fulfill.
Machine Learning
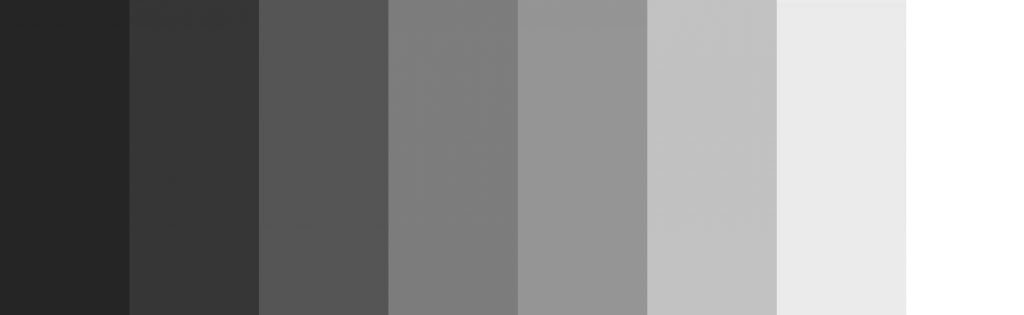
And there is the big thing missing between a human written blog article and something generated by AI. Human generated or AI generated is not binary. It is not one or the other. There is a whole greyscale between the black and the white of how to perform threat detections.
Yes the old school early 2000’s SIEMs needed a single use logic to find something precise in log entries. For the last 5+ years though, Machine Learning has been used for critical analysis and the detection of outliers and change. Businesses use Data Scientists to write their Threat Detections. People who understand how to tune data models and find anomalies, then being able to put context to each anomaly before defining it as a detection.
How AI (doesn’t actually) Redefines Threat Detection
If you believe the marketing spiel, AI-driven threat detection shifts the paradigm from predefined rules to anomaly detection. Instead of searching for specific patterns, AI models analyze vast amounts of data to identify deviations from normal behavior. This approach changes the focus from detecting what is known to spotting the unknown, potentially uncovering novel attack techniques or insider threats.
But this is incorrect. AI isn’t (or shouldn’t) being used to automate and optimise the way threat detections look for specific patterns. It should be used to tune and swap between the numerous algorithms for outlier detection.
Key characteristics of AI-driven detection include:
- Contextual Understanding: AI models learn the baseline behavior of systems, users, and networks, using this understanding to flag outliers.
- Threshold-Based Triggers: Instead of relying on a single rule, AI systems often combine multiple thresholds—such as unusual login times, unexpected data transfers, or geographic anomalies—to decide when human intervention is necessary.
- Continuous Learning: AI systems improve over time by incorporating feedback and adapting to new patterns.
Trusting AI in Threat Detection: Benefits and Challenges
Benefits:
- Scalability: AI can process and analyze vast amounts of data, far exceeding human capacity.
- Adaptability:
Unlike static rules, AI can evolve with changing threat landscapes. - Efficiency: By reducing false positives and focusing on significant anomalies, AI optimizes the workload for security teams.
Note the strike through. AI needs to learn, to understand when something should and shouldn’t be evolved. Yes it is more adaptable, but still needs human intervention to be directed away from its expected ‘norm’.
Challenges:
- Interpretability: AI decisions can sometimes lack transparency, making it harder to understand why a particular action was flagged.
- Trust Dependency: Trusting AI requires confidence in its training data, algorithms, and thresholds.
- False Negatives: Anomaly detection may overlook threats if they mimic normal activity too closely.
Trusting AI: A Comparative Perspective
Human-Written Detections
When a human writes a static detection rule, the process involves defining specific conditions to flag potential threats. For example, an analyst may create a rule that detects data exfiltration by flagging any file transfer exceeding 1GB within a short timeframe. This rule is precise and predictable, allowing for rigorous testing with both real and simulated data.
Strengths of human-written static detections include:
- Clear Logic: The conditions are explicit and understandable.
- Testability: Security teams can validate the detection’s effectiveness by injecting relevant data and observing consistent results.
- Immediate Feedback: Analysts can quickly tweak rules based on observed outcomes.
Machine Learning Detections
These detections operate differently. They rely on machine learning models that analyse patterns and detect anomalies across multiple dimensions. It makes no difference if they are human or AI written. It is all about the modelling and tuning of the envelope at which an incident fires.
Instead of focusing on predefined conditions, these look for unusual behavior, such as an employee logging in from two geographically distant locations within minutes.
The benefits of this approach include:
- Broader Coverage: Can detect subtle or novel threats that predefined rules might miss.
- Dynamic Adjustments: Models can adapt to new behaviors and refine their understanding over time.
- Reduced Noise: By correlating multiple anomalies, they minimizes false positives.
However, trusting AI instead of humans to write Machine Learning introduces complexities:
- Threshold Ambiguity: It’s not always clear which thresholds were exceeded or why.
- Limited Guarantees: Unlike human-written rules, AI does not guarantee consistent detection for specific scenarios.
Building Trust in AI-Based Threat Detection
To effectively transition to AI-driven threat detection, organizations must focus on fostering trust. This involves addressing concerns related to accuracy, reliability, and transparency.
1. Explainability
One of the primary challenges of trusting AI is understanding its decisions. Security teams need clear explanations of why an alert was (or wasn’t) triggered. This could involve:
- Visualizations: Providing graphical representations of anomalies and contributing factors.
- Root Cause Analysis: Explaining the thresholds and patterns that led to the detection.
2. Validation
Organizations must validate AI systems to ensure they function as intended. This includes:
- Testing with Real Data: Running AI models against real-world scenarios to assess performance.
- Simulating Threats: Injecting test data to verify that the system detects known attack patterns.
- Feedback Loops: Continuously improving AI models based on analyst input.
3. Hybrid Approaches
Combining human expertise with AI capabilities can enhance trust. For example:
- AI-Assisted Rules: Using AI to suggest or refine detection rules that analysts can review.
- Collaborative Alerts: Allowing AI to flag anomalies while humans decide on the appropriate response.
4. Governance and Accountability
Clear governance frameworks ensure that AI models align with organizational objectives and ethical standards. Key considerations include:
- Bias Mitigation: Ensuring training data represents diverse scenarios to avoid bias.
- Auditing: Regularly reviewing AI systems for accuracy and fairness.
- Accountability: Defining roles and responsibilities for managing AI-driven detections.
Trusting AI: A Strategic Imperative
Incorporating AI into SIEM tooling is not just a technological shift—it’s a strategic imperative. Trusting AI enables organisations to:
- Enhance Security Posture: Having removed the paragraph of why AI thinks AI improves security posture, I will admit it still does so -but not in the way it declared it did. In short it expedites the time to tune machine learning algorithms.
- Streamline Operations: Automated detections reduce the burden on security teams, allowing them to focus on higher criticality tasks.
Future-Proof Systems: Now unless AI sends John Connor back with a list of how the future will look, then it’s not going to be future proofing anything soon. We have no way to know what the role of Protective Monitoring is going to play in 5 years time, so any claim of future proofing just isn’t going to apply. However I commend the marketing teams for making this claim so often that when writing an article about AI, the business case ‘buzzword bingo’ allowed chatGPT to think this was true.
Case Study: Trusting AI in Action
Consider an organisation that implemented an AI-driven SIEM solution to enhance its cybersecurity operations. The AI system identified an unusual sequence of events:
- A user accessed sensitive files during non-business hours.
- The same user initiated a data transfer to an external server.
- The transfer occurred from an IP address not previously associated with the user.
Individually, these activities might not raise alarms. However, by correlating multiple anomalies, the AI flagged the behavior as suspicious. Upon investigation, the security team discovered unauthorised access by an external actor using compromised credentials.
But the AI has no context here. Maybe the business is highly complex and changes to production systems may not take place during office hours. It’s in the 2 to 3am change window and ‘Dan’ the junior engineer has logged into the file server from home. He’s been extra precautious and made sure that all the ‘fee earners’ documents have been closed safely and performed an extra ordinary backup to the cloud backup provider. With the backup verified, he then upgrades the server and logs back off.
This example underscores the value of trusting AI to detect complex, multi-faceted threats that human-written rules might overlook, or the AI over simplifying and over alerting when it has no business context.
Overcoming Barriers to Trusting AI
Building trust in AI requires overcoming common barriers:
Fear of Job Displacement
Some security professionals worry that AI will replace their roles. However, AI is not a replacement but a complement. It enhances human capabilities by automating repetitive tasks and providing deeper insights.
Personally, I see the implementation of AI as a Y2k event. A big thing will be made of it, loads will be spent on over complicating its deployment and the only people who have an issue will be those who either do nothing at all, or just don’t take a valid approach to deploying it in a cost effective manner.
Resistance to Change
Transitioning from human-written rules to machine learning driven systems involves cultural and operational changes. Organisations can ease this transition by:
- Providing Training: Equipping teams with the skills to work with big data.
- Highlighting Benefits: Demonstrating how data analysis improves efficiency and effectiveness.
Concerns About Reliability
Skepticism about AI’s reliability can hinder adoption. Addressing these concerns involves:
- Proving Value: Showcasing real-world success stories.
- Continuous Monitoring: Regularly evaluating AI performance to ensure consistent results.
Conclusion
Trusting AI in threat detection is not merely about adopting new technology—it’s about embracing a paradigm shift. While human-written detections offer precision and predictability, AI-driven systems provide adaptability and could provider broader threat coverage. By addressing challenges related to transparency, validation, and cultural acceptance, organisations can harness the full potential of AI.
As cyber threats continue to evolve, CISO’s require an essential strategy for staying ahead and need to communicate their appetite in trusting AI.
The other conclusion is also that I could probably have written this article quicker than using AI.
View Comments
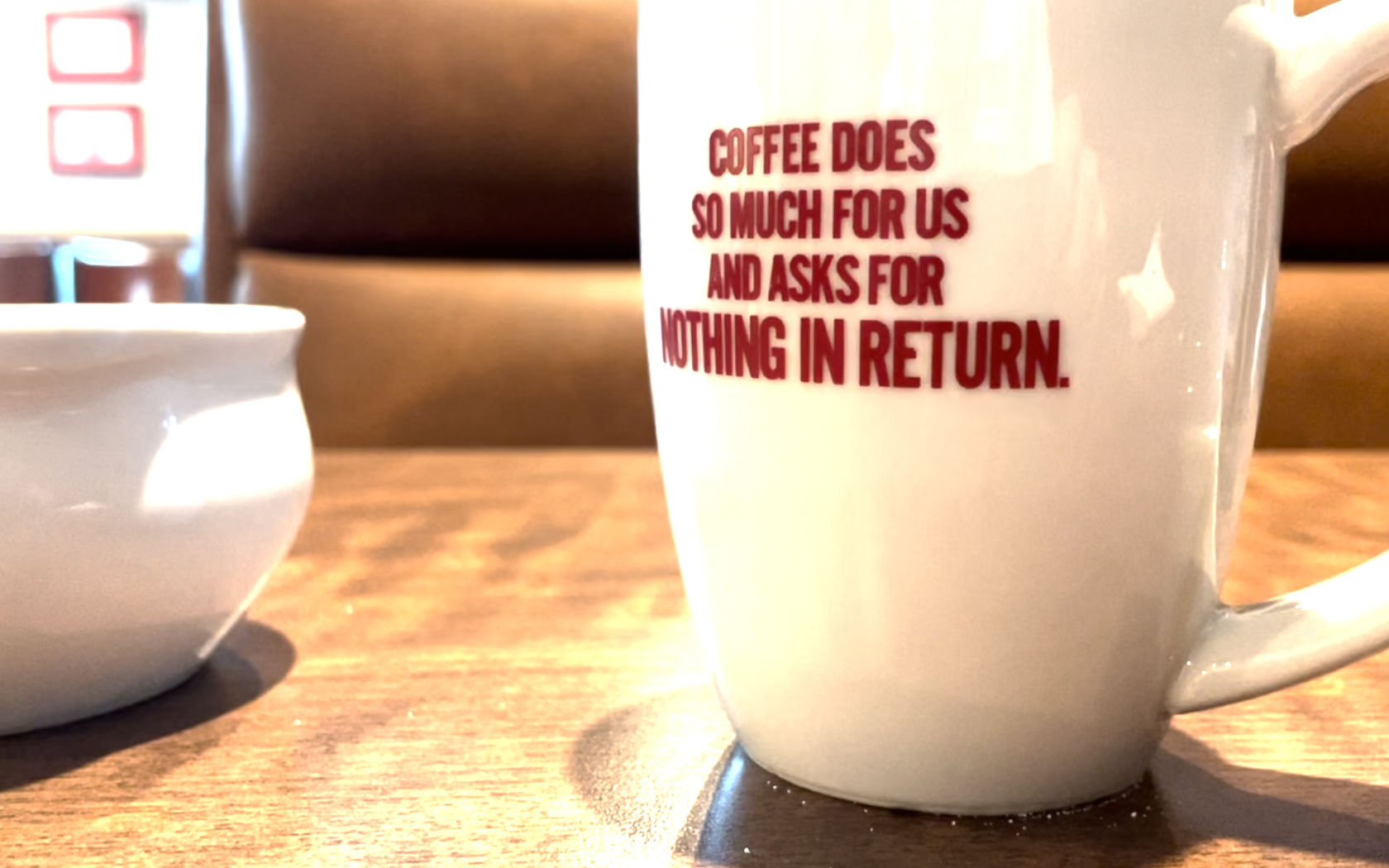
The Future of SIEM Platforms: From Obsolescence to Renaissance
This blog explores the Future of SIEM Platforms and their evolving role in modern...